Supporting data users across the Yale medical campus through instruction, collaboration, and consultation
Get in Touch
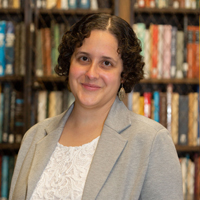
Kaitlin Throgmorton, MLIS
Data Librarian for the Health Sciences
kaitlin.throgmorton@yale.edu
Frequently Asked Questions
What are research data?
Definitions abound, but one way to define research data is raw outputs resulting from the process of research and inquiry, such as:
- Experimental data
- Clinical and demographic data
- Survey data
- Mined text
- Images
- Qualitative data, such as interview transcripts, diaries, field observations, etc.
References:
- Australian National Data Service. (2017). What is research data. https://ardc.edu.au/
- Borgman, C.L. (2015). Big data, little data, no data: Scholarship in the networked world. MIT Press.
- Byrd, J.B., Greene, A.C., Prasad, D.V. et al. (2020). Responsible, practical genomic data sharing that accelerates research. Nature Reviews Genetics 21, 615–629. https://doi.org/10.1038/s41576-020-0257-5
- National Institutes of Health. (n.d.). Research Covered by the 2023 Data Management & Sharing Policy: Scientific Data.
Where should I store my data?
Use this handy storage finder tool to determine the best Yale-provided data storage solution for your needs. As Yale simplifies some of its storage options, we encourage you to monitor these updates on the ITS Collaboration Counts page.
If you need to compute against your data, contact the Yale Center for Research Computing.
Where can I find data to reuse for an assignment, project, or research study?
Data can be found in many places, such as in library databases (e.g., PubMed) as supplementary materials in journal articles and in data repositories.
Check out our "Find Data" and "Re(Use) Data" guides to learn more.
What’s research data management?
Research data management is the care and maintenance of data produced during research. It starts when your project starts, and continues through the end of the project, and sometimes extends beyond that. It has many components, but in summary, it involves planning, organizing, documenting, storing, securing, assessing, citing, and sharing your data alongside your research.
Learn more on our "Manage Data" page.
What are Yale’s expectations for how I manage my data?
Review Yale’s Research Data and Materials Policy (6001), and ensure you know your data’s classification status — you can take this questionnaire to find out. Whether your data is low, medium, or high risk determines where you can store it, who can use it (and how), and how you should manage it.
Additionally, if applicable, review other standards and requirements, such as HIPAA and IRB policies.
Learn more on our "Manage Data" page.
I want to learn skills to better work with my data. Where should I start?
Many people learn programming languages such as Python and R to work with data. Others use software, such as Excel, other spreadsheet applications (e.g., LibreOffice Calc, Google Sheets), SPSS, SAS, Tableau, and many others.
In addition to resources at and provided by Yale (including classes at the medical library, on LinkedIn Learning, and at other places on campus, such as the StatLab, Yale Center for Biomedical Data Science, and Yale Center for Research Computing), view more recommended resources on our "Learn to Work with Data" page.
Upcoming Training